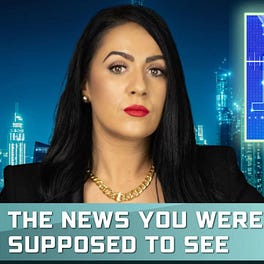
One of the buzzwords of 2025 is going to be βWorld Modelsβ. Numerous well-funded companies have already announced that theyβre building them, and established players have made it clear that theyβre in the race too. But what are they?
Iβve found myself explaining why World Models are important and how they work to many friends who either arenβt techies or who work in tech fields other than 3D, and I thought to write the explanation I usually give them but as a blog post so that other people can also access it.
Iβm purposely going to stay high-level. I hope to reach people who are looking to either investigate / invest in companies doing this but donβt need to know the nitty gritty details, and give them a mental framework for how to think about World Models so that they can then go and investigate on their own. Iβm going to make simplifications along the way in that spirit.
Another important note: World Models can be used for more than generating 3D worlds or generating more consistent videos (more on that later). For example, the term can be used to describe models that understand the real world from images, videos, and LiDAR, and take action based on that. For that purpose, video-based supervision is not enough, and we need more data about interaction with the environment.
But in this article, Iβll focus on World Models for the purpose of generating data, not physics simulation or robotics navigation. This is because my background is in 3D vision and 3D graphics, and describing World Models from that angle is what Iβve found to be a great way to explain it to others too, and the way I think about them.
Iβll leave further reading at the end for whoever wants to go deeper and also build a technical understanding.
In the beginning there was NeRF
In order to tell the full story of World Models, we have to go back to 2020, when a technique called NeRF (Neural Radiance Fields) came out. This is because NeRF played (plays?) a role in how world models came about.
There are already many good articles that cover NeRF, so Iβm not going to explain it in detail here. A great starting point is https://dellaert.github.io/NeRF/
But in a nutshell, NeRF is a way to reconstruct a 3D scene using Machine Learning (more specifically, training a neural network parameterized representation) rather than using handcrafted algorithms, achieving a level of realism that was hard/impossible to achieve before.
My favorite analogy to explain NeRFβs importance goes like this: before Convolutional Neural Networks, researchers would spend time handcrafting matrices for feature detection in images: theyβd literally be filling out matrices with numbers by hand, and then using those for image-related tasks (say, edge detection).
But when ConvNets came about, doing that by hand became obsolete: the machine would instead learn autonomously the optimal weights to use in the feature detection matrices. You just needed to feed it enough data. And the weights it produced were much better at feature detection than the handcrafted ones found by humans.
In the same vein, NeRF can be thought of as going from handcrafted data representations (e.g. 3D meshes) to asking the machine to learn how to represent a 3D scene by just showing it the data.
We then got a large amount of variants of NeRF that optimized for speed, quality, and ease of use, leading eventually to Gaussian Splats, which by now have effectively replaced NeRF as the go-to tool for 3D neural reconstruction.
A key factor is that in NeRF and variants youβre **overfitting a model** to a specific scene: the model youβve trained isnβt generalizable, i.e. you canβt ask it to generate a different scene from the one you overfit it on.
(π‘ Iβm well aware that we now have feed-forward methods for neural 3D scene reconstruction that donβt overfit to a scene. Iβm simplifying here and taking the historical route to put this all into context.)
This eventually created an appetite for not only neural reconstruction of a single scene, but also for generating 3D scenes and assets using neural approaches. And not only static 3D scenes, but dynamic ones, too!
However, the solution for how to achieve this would be found not in 3D, but in 2D.
Video Models
While all of this was going on, other companies & researchers had been working on image generation, and more recently on video generation. I bet by now many of you are familiar with video generation models, such as Sora from OpenAI or Mochi from Genmo AI, so this section should sound familiar.
Put simply, these models work by producing a series of video frames starting from the userβs text prompt and, sometimes also taking into account a starting frame and end frame. Think of them like an image diffusion model, but one thatβs been specialized to produce video frames, in the time dimension.
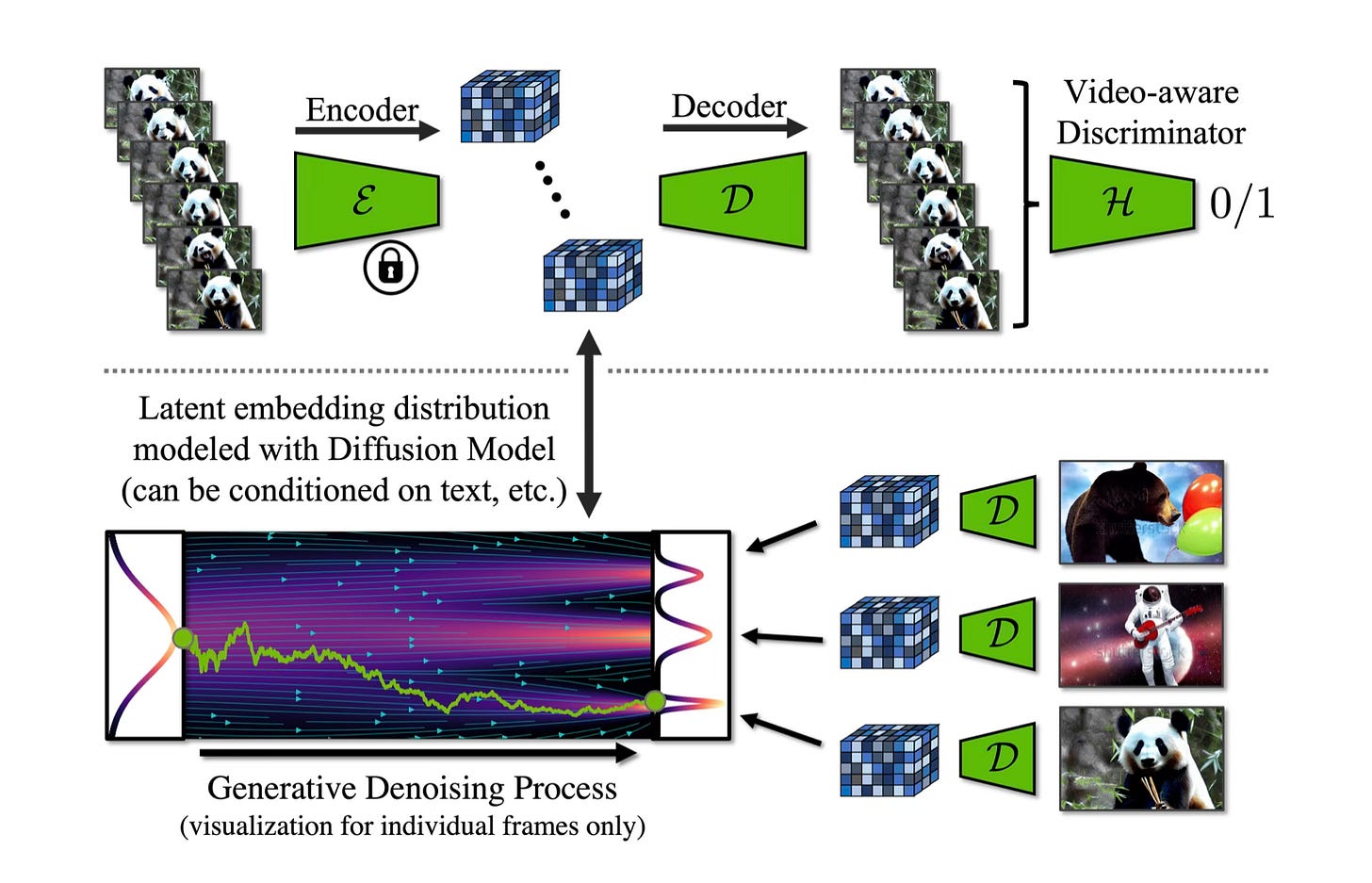
Compared to NeRFs and its spinoffs, video and image generation requires a lot of data in order to become useful. These generative methods aim to learn the true distribution of the data they are sampling from, which needs to be as broad as possible to enable the expressivity required to generate diverse videos or images. Essentially, you're asking the model to learn to draw by showing it extensive examples, fitting it to the underlying data distribution without overfitting, so creativity is preserved.
In contrast, NeRFs operate more like regression tasks. They leverage extensive pixel-level supervision, fitting a model to match specific scenes with high fidelity. This distinction underscores the limitations of video supervision alone.
In fact, another important difference between NeRF/GS and text/image/video β image/video/3D generation models is that:
in the former case, like we said above, youβre overfitting a model to a specific, small dataset
in the latter, youβre learning from a large dataset and creating a generalized, feed-forward model that can produce a variety of new outputs.
For the task of learning to produce images or videos, we have a ton of data to use from the internet. But for 3D generation, we donβt have that much 3D native data to use for learning. For comparison, the largest dataset of 3D objects, Objaverse-XL, is 10M assets. Compare that to the datasets used by image and video companies today (e.g. LAION, which is 5B), and you can see that the difference is stark. Without enough 3D data, training models to generate 3D by learning from 3D examples is hard.
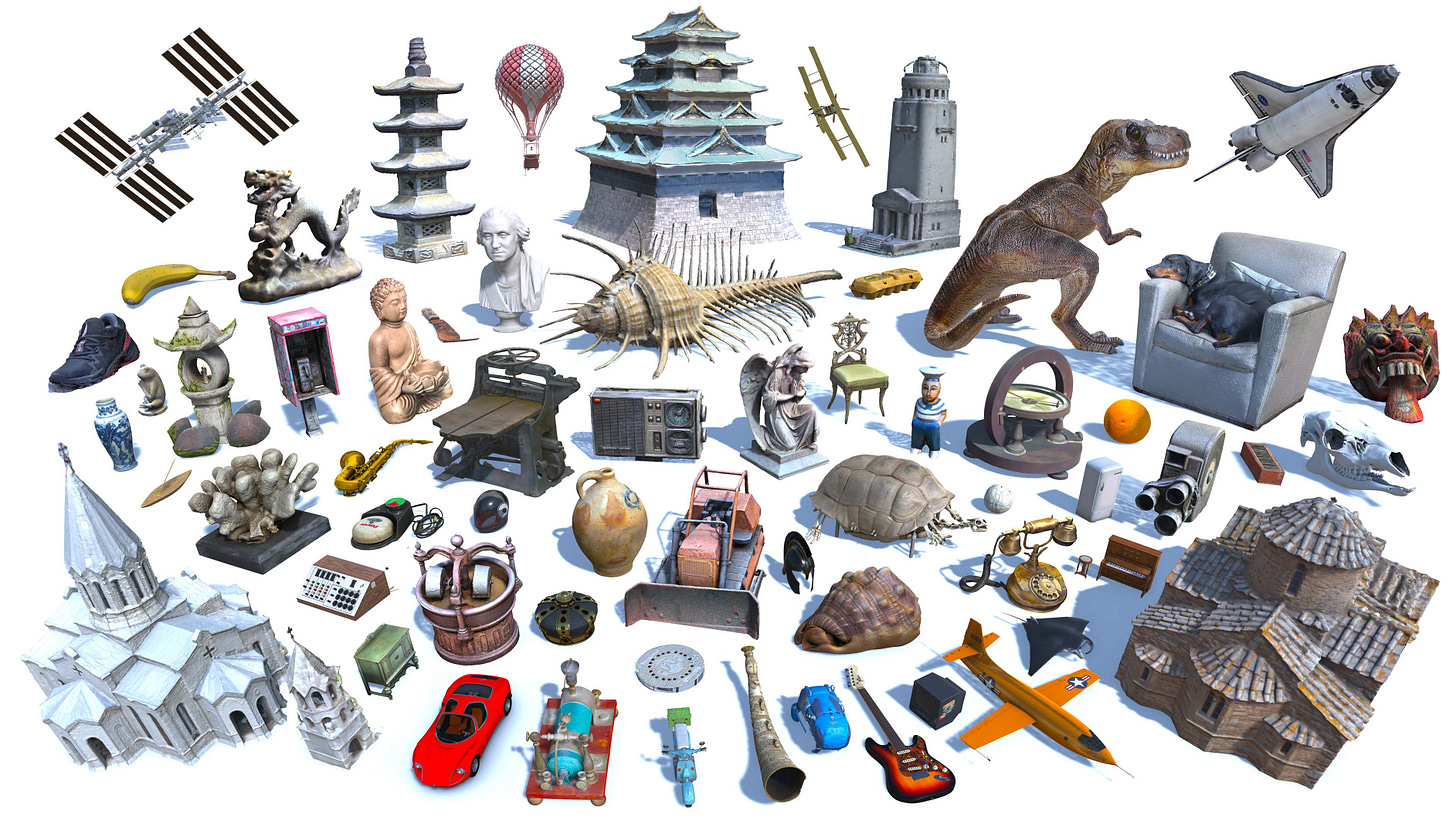
Interestingβ¦ Weβd have to show this model a lot of video data, and ask it to learn to make predictions (i.e. generate some kind of output) based on that data. Sound familiar?
Thatβs basically what you have to do to train video models!
3D Rendering
Now keep that in mind, and switch for a second to thinking about how videogames are displayed to you when you play them: thereβs a 3D rendering engine that takes a 3D scene, and renders it to a 2D screen that you can then look at.
A 3D engine does this frame by frame, N times a second, creating the illusion of a real-time experience. As you move around the 3D scene, itβs going to render this 3D scene from different perspectives, allowing you to explore it, and view more of it.
Notice how when I look away from the tree, then look at it again, itβs the same tree that I was looking at before.
What matters here is that as you navigate through the scene, if you look at object A, then look away, and then look at it again, youβll see the same object A, not something different. I.e. there is βobject permanenceβ.
Video models also have to kind-of render (or predict?) the next 2D frame in a sequence of frames. But most importantly, they also have to learn certain βpriorsβ in order to work well:
if I look at an object from different angles, the object should remain the same, not be hallucinated
an object moving across the screen should remain the same object across frames, not morph into something else
We can call this ability βMultiview Consistencyβ. And what this does sounds awfully familiar to what you get βfor freeβ from a 3D scene being rendered (see the demo above again) with canonical rendering methods, in 3D engines.
So, what if we could take a video model, and:
run it in real-time, like a 3D engine
steer it in real-time, like when you control a camera in a 3D engine
improve how realistic it looks (Multiview Consistency) so much that as you look at the same object across time, that object doesnβt change (you guessed it, like in a 3D engine)
?
If that were possible, and you used such model, you wouldnβt know the difference between running that, or playing a game in 3D engine which is rendering a 3D scene!
World Models
So now we can finally give our first definition of what a World Model is: it's a model that can generate the next frame of a 3D scene based on the previous frame(s) and user input, trained on video data, and running in real-time.
The key insight is that by training on video data, these models learn not just how to generate images, but also:
the physics of our world (objects fall down, water flows, etc)
how objects look from different angles (that chair should look the same as you walk around it)
how things move and interact (a ball bouncing off a wall, a character walking on sand)
basic spatial understanding (you can't walk through walls)
And they learn all of this on their own, just by watching videos. This is cool because traditionally, to create a 3D world with physics, you'd need to explicitly program all these rules in.
There are a bunch of implications to this. Imagine if you could:
generate entire virtual worlds on the fly just by describing them in text
create complex physics simulations without writing a single equation
design virtual movie sets that directors can explore in real-time
build video games where every playthrough generates a completely unique world
So if their promise is fulfilled, the next years are going to be interesting.
Current examples
Recently, Decart came out with Oasis, their demo world model that they taught to simulate and render Minecraft:
Decartβs Oasis, via @_akhaliq
And you can actually try it today! I want to stress that in these demos, there isnβt a 3D engine involved. The model has learnt what it means to render minecraft given your inputs and the previous frames, and it predicts what should happen the next frame.
Another example is DIAMOND, by the University of Geneva, the University of Edinburgh, and Microsoft Research:
They taught it to simulate and render another videogame, Counter Strike: GO. You can try this one too!
And other companies have all announced their version: Google, RunwayML, Meta, Wayve, & more.
There are still significant challenges to overcome. Running these models in real-time requires a lot of computational power. And while they're getting better at maintaining consistency (making sure that chair stays the same as you walk around it), they're not yet perfect.
Why we looked at NeRF to begin with
This is why some companies, like World Labs, are taking a hybrid approach: using World Models to generate static 3D representations that can then be rendered using traditional 3D engines (in this case, Gaussian Splatting). This gives you the best of both worlds: the creative power of AI generation with the multiview consistency and performance of traditional rendering.
A demo of World Labsβs product by Martin Casado
Odyssey, who recently also entered the World Models race, are also doing something similar, producing a Gaussian Splats scene:
You can see how in a way weβve come full circle: from NeRF, to Gaussian Splats, to Video Models, to World Models, back to Gaussian Splats (NeRFβs youngest offspring).
Looking ahead
As computational power increases and these models continue to improve, we're likely to see World Models (or whatever spinoff we get from them) become a fundamental technology in how we create and interact with virtual environments. They represent a paradigm shift from manually crafted 3D worlds to AI-generated ones that understand and can simulate the physics and logic of our reality.
As Yann LeCun emphasized in 2022, interaction with the environment is key for advancing generative methods. Models need to move beyond static video data, integrating dynamic environmental interaction to develop richer, more accurate representations of the world. For this reason, in time weβll see World Models more and more used outside of games and video generation.
But we're just at the beginning, and the applications we've discussed are just the tip of the iceberg, and I expect we'll see use cases emerge that we haven't even thought of yet throughout 2025 and onwards.
Edit: Things are moving fast
And just today (Dec 18 2024), a large research group released GENESIS: a ββ¦comprehensive physics simulation platform designed for general purpose Robotics, Embodied AI, & Physical AI applications. It is simultaneously multiple things:
A universal physics engine re-built from the ground up, capable of simulating a wide range of materials and physical phenomena.
A lightweight, ultra-fast, pythonic, and user-friendly robotics simulation platform.
A powerful and fast photo-realistic rendering system.
A generative data engine that transforms user-prompted natural language description into various modalities of data.β
And itβs open source!
Althought this is not exactly a World Model in the way described in this article, itβs going to generate a lot of interest in the subject. Expect papers on the subject.
Further reading
If you want to dive deeper into the technical aspects of World Models, here are some resources:
the original NeRF paper
the original 3D Gaussian Splatting paper which took the 3D world by storm
various implementations and approaches by research labs and companies working on World Models (see X links below)
https://x.com/weichiuma/status/1866922624598806900
https://x.com/GoogleDeepMind/status/1864367798132039836
https://x.com/___Harald___/status/1866345938639548513
https://x.com/_akhaliq/status/1834590455226339492
https://x.com/DecartAI/status/1852091173420294291
https://x.com/theworldlabs/status/1863617989549109328
https://x.com/EloiAlonso1/status/1844803606064611771
https://x.com/wayve_ai/status/1759951847270355336
https://x.com/runwayml/status/1734212913936666750
https://x.com/EloiAlonso1/status/1866931236171653401
https://x.com/wenhaocha1/status/1864512918634811653
Many thanks to Cosimo Taiuti, Alex Kristoffersen and Alex Bergman for reviewing it.