Recommending related products — say, a phone case to go along with a new phone — is a fundamental capability of e-commerce sites, one that saves customers time and leads to more satisfying shopping experiences.
At this year’s European Conference on Machine Learning (ECML), my colleagues and I presented a new way to recommend related products, which uses graph neural networks on directed graphs.
In experiments, we found that our approach outperformed state-of-the-art baselines by 30% to 160%, as measured by hit rate and mean reciprocal rank, both of which compare model predictions to actual customer co-purchases. We have begun to deploy this model in production.
The main difficulty with using graph neural networks (GNNs) to do related-product recommendation is that the relationships between products are asymmetric. It makes perfect sense to recommend a phone case to someone who’s buying a new phone but less sense to recommend a phone to someone who’s buying a case.
A graph can capture that type of asymmetry with a directed edge, which indicates that the relationship between two graph nodes flows in only one direction. But directedness is hard for GNN embeddings — that is, the vector representations produced by GNNs — to capture.
We solve this problem by producing two embeddings of every graph node: one that characterizes its role as the source of a related-product recommendation and one that characterizes its role as the target. We also present a new loss function that encourages related-product recommendation (RPR) models to select products along outbound graph edges and discourages them from recommending products along inbound edges.
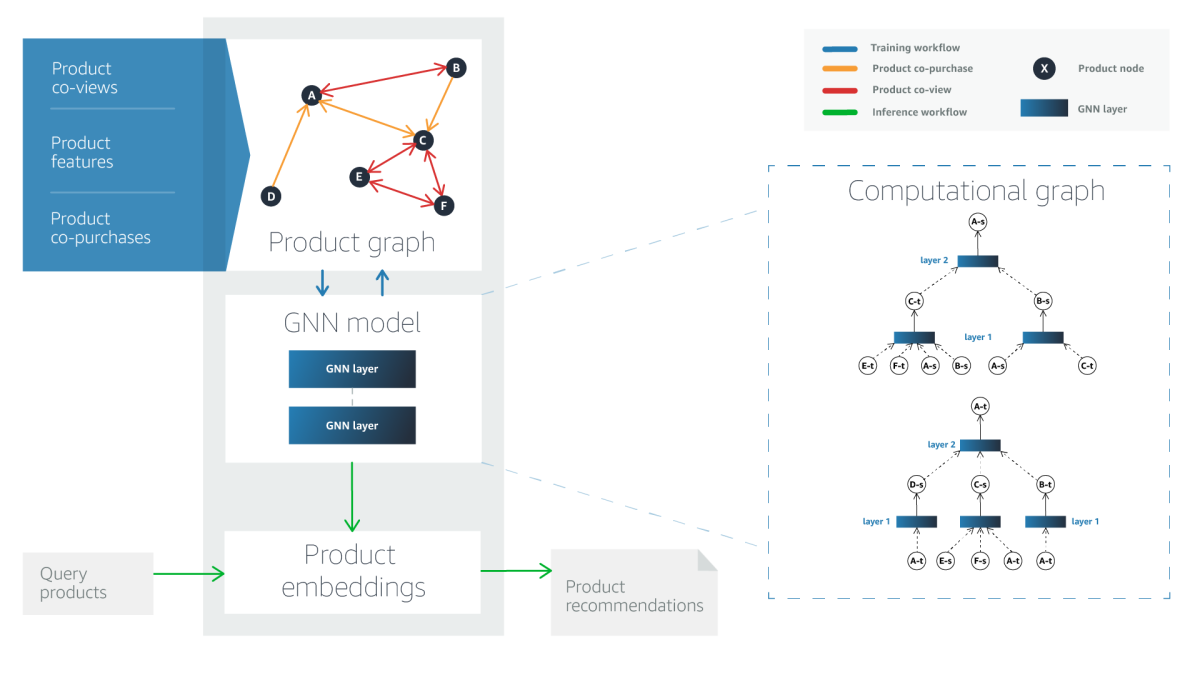
Because our GNN takes product metadata as an input — as well as the graph structure — it also helps address the problem of cold start, or how to account for products that have only recently been introduced to the catalogue. Finally, we introduce a data augmentation method that helps overcome the problem of selection bias, which arises from disparities in the way information is presented.
Graph building
In our product graph, the nodes represent products, and the node data consists of product metadata — product name, product type, product description, and so on. To add directional edges to the graph, we use co-purchase data, or data on which products tend to be purchased together. These edges may be unidirectional, as when, say, one product is an accessory of another, or bidirectional, if products are co-purchased, but neither depends on the other.
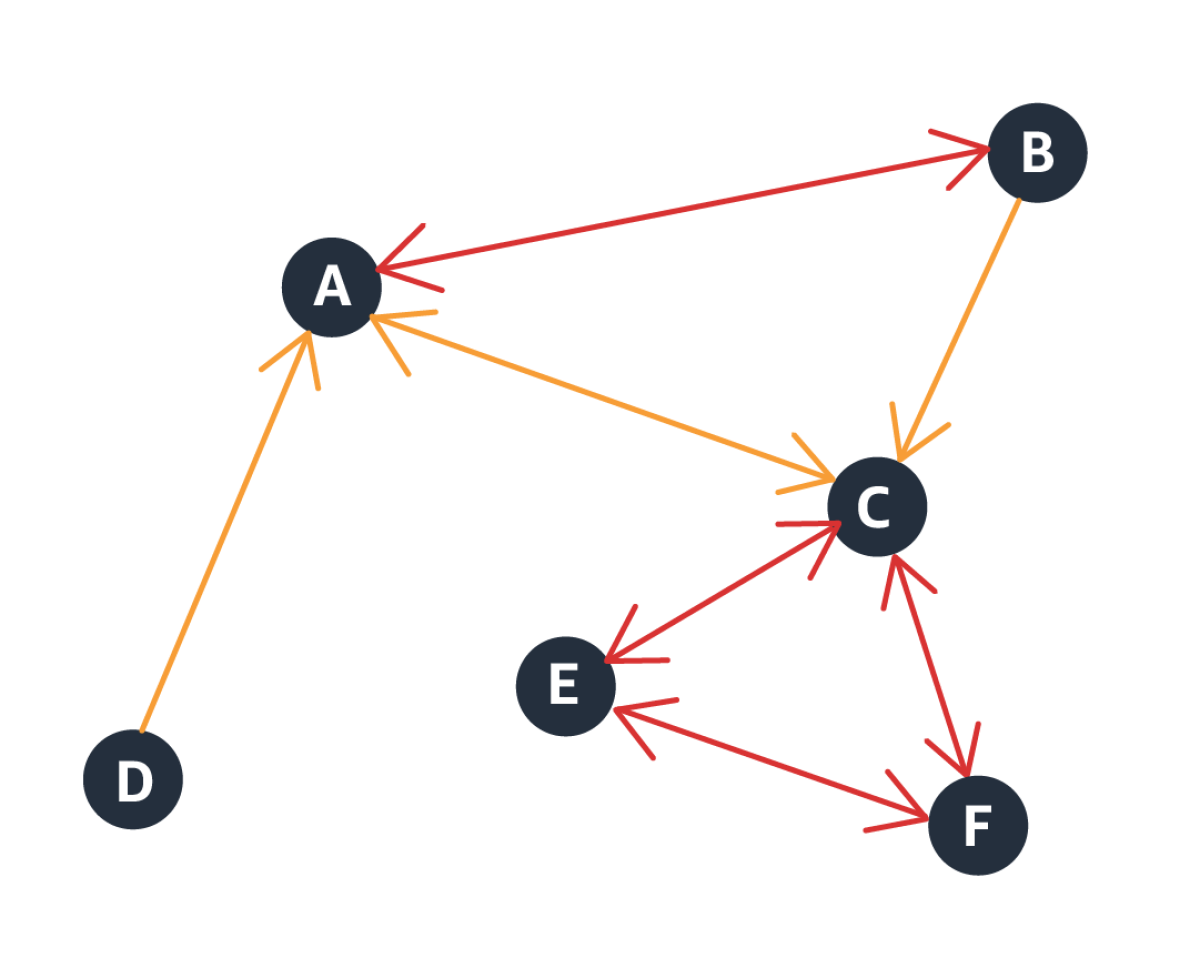
This approach, however, runs the risk of introducing selection bias into the model. In this context, selection bias occurs when customers’ preferential selection of one product reflects greater exposure to that product. To offset that risk, our graph also includes bidirectional edges that we derive from co-view data, or data on which products tend to be viewed together under the same product query. Essentially, the co-view data helps us identify products that are similar to each other.
The product graph thus has two types of edges: edges indicating co-purchases and edges indicating similarity.
GNN embeddings
For each node in the product graph, the GNN produces an embedding, which captures information about the node’s immediate vicinity. We use two-hop embeddings, meaning they factor in information about both a node’s immediate neighbors and those nodes’ neighbors.
The key to our model is the procedure for generating separate source and target embeddings. For each node, the source embedding factors in all the node’s similarity relationships but only its outbound co-purchase relationships. Contrarily, the target embedding factors in all the node’s similarity relationships but only the inbound co-purchase relationships.
The GNN is multilayered, and each layer takes in the node representations produced by the layer below and outputs new node representations. At the first layer, the representations are simply the product metadata, so the source and target embeddings are the same. Beginning at the second layer, however, the source and target embeddings diverge.
Thereafter, the source embedding for each node factors in the target embeddings of the nodes with which it has outbound co-purchase relationships and the source embeddings of the nodes with which it has similarity relationships. The target embedding for each node factors in the source embeddings of the nodes with which it has inbound co-purchase relationships and the target embeddings of the similar nodes.
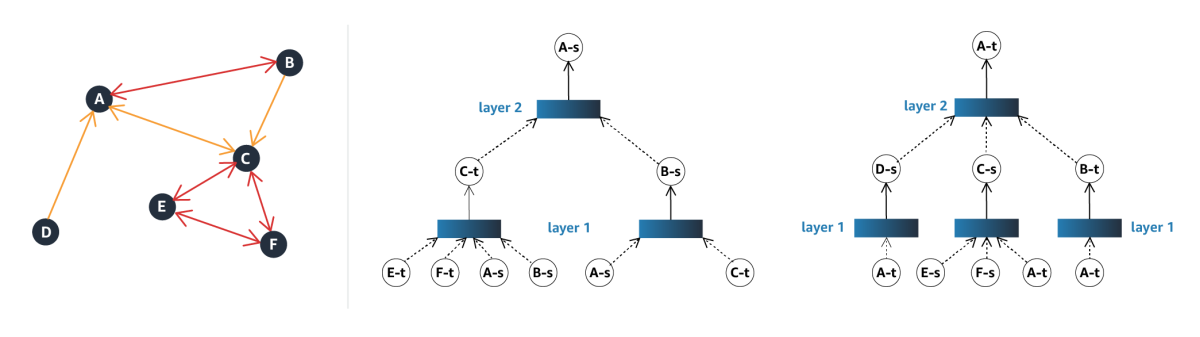
We train the GNN in a self-supervised way using contrastive learning, which pulls the embedding of a given node and those that share edges with it together, while pushing apart the embedding of the given node and a randomly selected, unconnected node. A term of the loss function also enforces the asymmetry in the source and target embeddings, promoting the incorporation of information about target nodes connected by outbound edges and penalizing the incorporation of information about target nodes connected by inbound edges.
Once the GNN is trained, selecting the k best related products to recommend is simply a matter of identifying the k nodes closest to the source node in the embedding space. In experiments, we compared our approach to its two best-performing predecessors, using hit rate and mean reciprocal rank for the top 5, 10, and 20 recommendations, on two different datasets, for 12 experiments in all. We found that our method outperformed the benchmarks across the board — often by a large margin. You can find more details in our paper.