In the digital era, when documents are generated and distributed at unprecedented rates, automatically understanding them is crucial. Consider the tasks of extracting payment information from invoices or digitizing historical records, where layouts and handwritten notes play an important role in understanding context. These scenarios highlight the complexity of document understanding, which requires not just recognizing text but also interpreting visual elements and their spatial relationships.
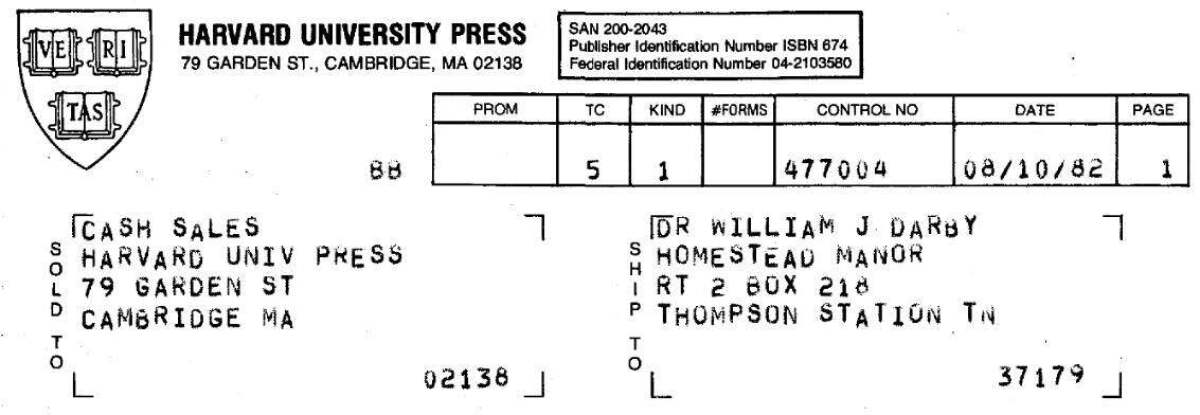
At this year’s meeting of the Association for the Advancement of Artificial Intelligence (AAAI 2024), we proposed a model we call DocFormerv2, which doesn't just read documents but understands them, making sense of both textual and visual information in a way that mimics human comprehension. For example, just as a person might infer a report's key points from its layout, headings, text, and associated tables, DocFormerv2 analyzes these elements collectively to grasp the document's overall message.
Unlike its predecessors, DocFormerv2 employs a transformer-based architecture that excels in capturing local features within documents — small, specific details such as the style of a font, the way a paragraph is arranged, or how pictures are placed next to text. This means it can discern the significance of layout elements with higher accuracy than prior models.
A standout feature of DocFormerv2 is its use of self-supervised learning, the approach used in many of today’s most successful AI models, such as GPT. Self-supervised learning uses unannotated data, which enables training on enormous public datasets. In language modeling, for instance, next-token prediction (used by GPT) or masked-token prediction (used by T5 or BERT) are popular.
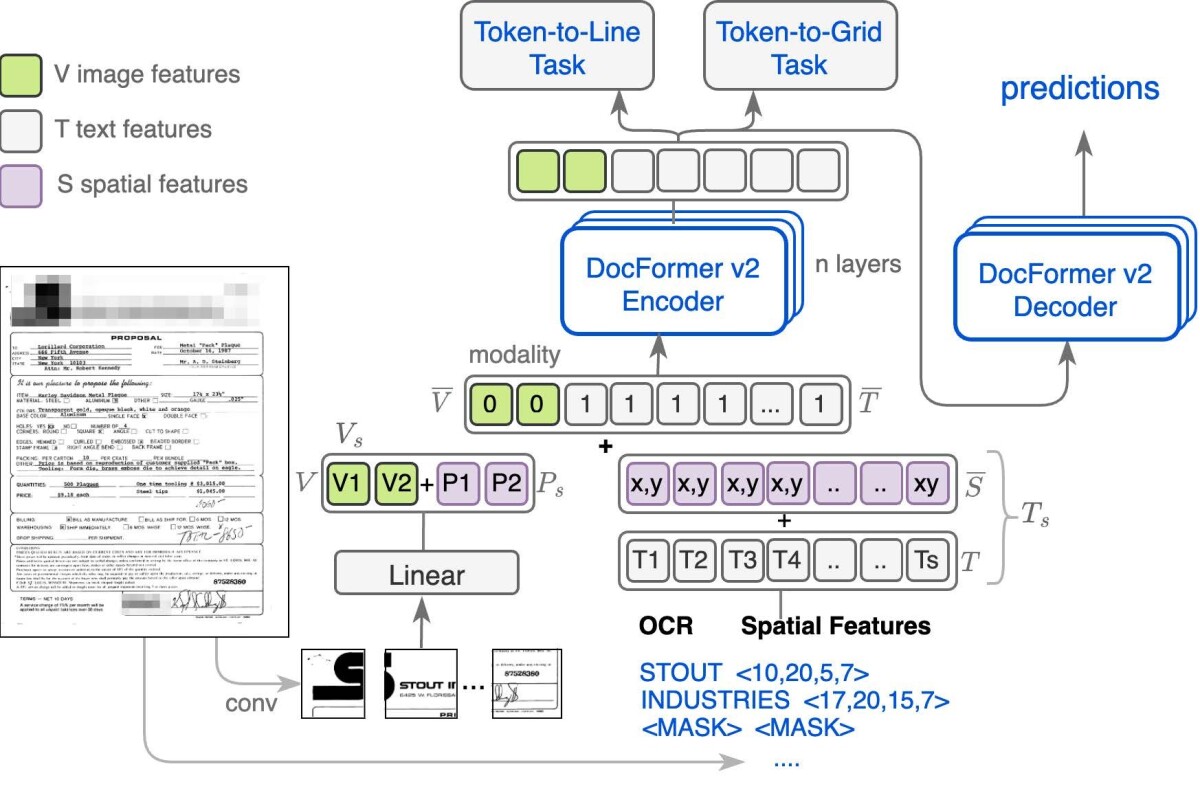
For DocFormerv2, in addition to standard masked-token prediction, we propose two additional tasks, token-to-line prediction and token-to-grid assignment. These tasks are designed to deepen the model's understanding of the intricate relationship between text and its spatial arrangement within documents. Let’s take a closer look at them.
Token to line
The token-to-line task trains DocFormerv2 to recognize how textual elements align within lines, imparting an understanding that goes beyond mere words to include the flow and structure of text as it appears in documents. This follows the intuition that most of the information needed for key-value prediction in a form or for visual question answering (VQA) is on either the same line or adjacent lines of a document. For instance, in the diagram below, in order to predict the value for "Total" (box a), the model has to look in the same line (box d, "$4.32"). Through this type of task, the model learns to give importance to information about the relative positions of tokens and its semantic implications.
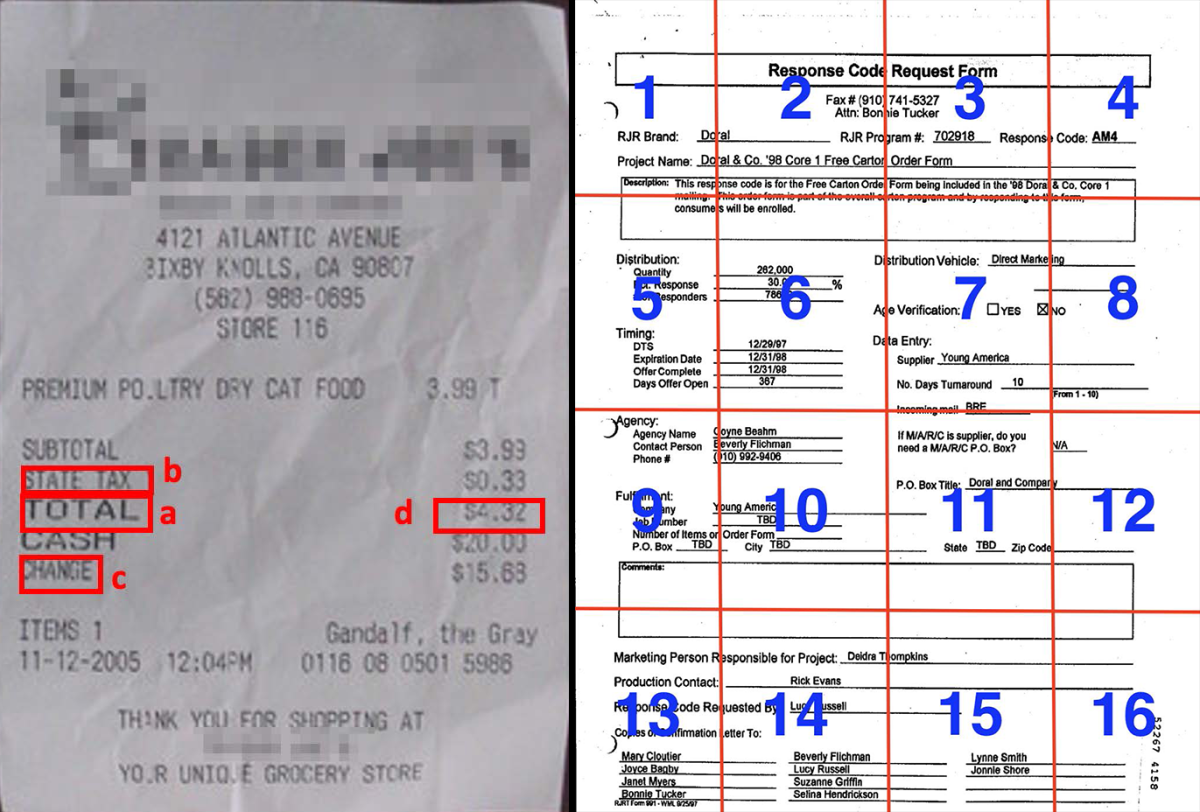
Token to grid
Semantic information varies across a document's different regions. For instance, financial documents might have headers at the top, fillable information in the middle, and footers or instructions at the bottom. Page numbers are usually found at the top or bottom of a document, while company names in receipts or invoices often appear at the top. Understanding a document accurately requires recognizing how its content is organized within a specific visual layout and structure. Armed with this intuition, the token-to-grid task pairs the semantics of texts with their locations (visual, spatial, or both) in the document. Specifically, a grid is superimposed on the document, and each OCR token is assigned a grid number. During training, DocFormerv2 is tasked with predicting the grid number for each token.
Target tasks and impact
On nine different datasets covering a range of document-understanding tasks, DocFormerv2 outperforms previous comparably sized models and even does better than much larger models — including one that is 106 times as big as DocFormerv2. Since text from documents is extracted using OCR models, which do make prediction errors, we also show that DocFormerv2 is more resilient to OCR errors than its predecessors.
One of the tasks we trained DocFormerv2 on is table VQA, a challenging task in which the model must answer questions about tables (with either images, text, or both as input). DocFormerv2 achieved 4.3% absolute performance improvement over the next best model.


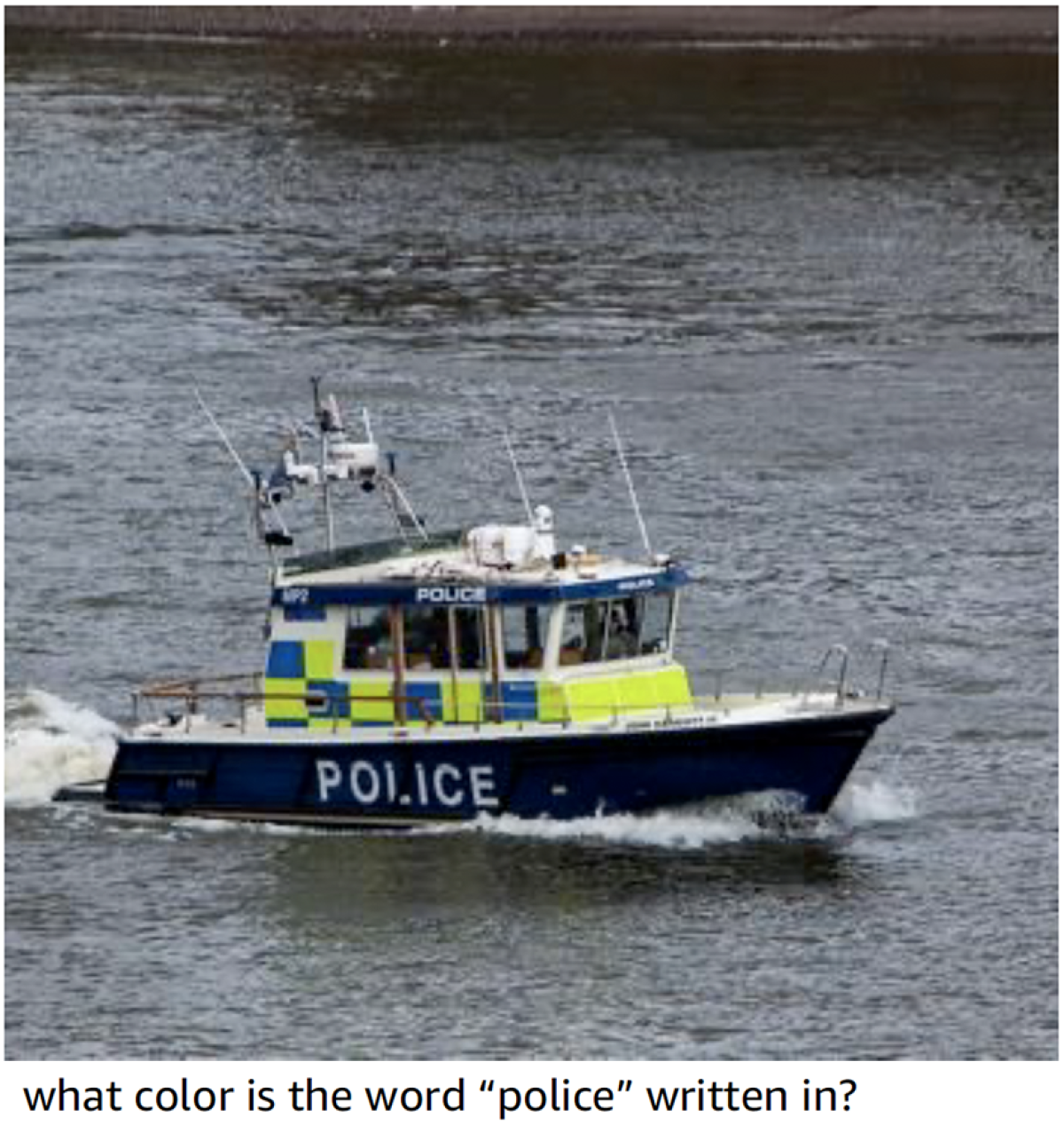
But DocFormerv2 also displayed more-qualitative advantages over its predecessors. Because it’s trained to make sense of local features, DocFormerv2 can answer correctly when asked questions like “Which of these stations do not have a ‘k’ in their call sign?” or “How many of the schools serve the Roman Catholic diocese of Cleveland?” (The second question requires counting — a hard skill to learn.)
In order to show the versatility and generalizability of DocFormerv2, we also tested it on scene-text VQA, a task that’s related to but distinct from document understanding. Again, it significantly outperformed comparably sized predecessors.
While DocFormerv2 has made significant strides in interpreting complex documents, several challenges and exciting opportunities lie ahead, like teaching the model to deal with diverse document layouts and enhancing multimodal integration.