Share this discussion
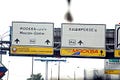
Comment by Maximum Limelihood Estimator on Astral Codex Ten
Hi Scott; it's the inverse-square-root. The standard error of an estimate declines as a function of…
284 Comments
© 2024 Scott Alexander
Substack is the home for great culture
Hi Scott; it's the inverse-square-root. The standard error of an estimate declines as a function of 1 / sqrt(n) for sample size n (because the variance declines with 1/n).
If the estimates are biased, the root-mean-square error is going to be sqrt(bias^2 + (variance / n)) for sample size n, i.e. the mean squared error will decline hyperbolically. This isn't something the study found; it's a mathematically-derived formula, which they then fit to the data to get estimates for bias^2 and variance. Because estimates taken from 1 person are going to be substantially biased, the error will never reach 0; it asymptotes out very quickly. The average of many people is going to be much less biased, such that the variance probably dominates.